Master data forms the foundation for almost all business processes, so a lack of quality can have serious consequences for value-adding processes and reporting. A well-founded master data strategy and the targeted use of tools can improve the initial situation. However, more is needed to benefit from high data quality in the long term.
Incomplete or incorrect master data delays downstream processes and causes them to run inefficiently. The best example of this is the creation of the material master: the material master is a highly complex and integrative master data object that is used by many departments. If, for example, the material is not maintained correctly when certain materials are initially created, this leads to problems in customs tariff classification. This makes the shipping process unnecessarily urgent and time-consuming, as the correct information has to be obtained under time pressure.
The material master is only one example of this; for critical objects, poor master data quality affects almost all areas of a company, from the finance department to marketing. In contrast, high quality master data offers advantages such as increased efficiency in business processes, greater reliability in forecasting and improved reporting. High-quality master data also helps to exploit the potential of digitalisation and use new technologies more effectively. In today’s data-driven business world, the quality of master data is a strategic factor for the long-term success of a company.
Master data in the SAP S/4HANA transition
It is always the right time to tackle master data quality. However, in some situations there is no way around it – for example, when integrations are pending as part of merger & acquisition activities. Companies often only systematically tackle the issue of master data quality as part of the SAP S/4HANA transformation. The full extent of the master data problems are then recognized in the preliminary studies for the transformation, which we refer to as the “uncovering effect”. This becomes apparent with regard to customer and supplier master data, for example, when the SAP Business Partner is introduced which is a central master data object under S/4HANA and combines various business functions. However, certain quality requirements must be met before the Business Partner can be introduced. cbs uses its own analysis tool, cbs MDV, to check the current master data for business partner suitability. Our tool also enables us to track the progress of the clean-up afterwards.
The S/4HANA transformation offers a valuable opportunity for harmonisation and standardisation, especially if selective transformation approaches are chosen. The recommendation is to take advantage of this moment, but also to anchor the topic of master data quality as a continuous endeavour within the company.
Get Data Clean: How can data quality be improved?
Individual initiatives are usually not enough to fundamentally improve processes and data quality. A master data strategy is therefore recommended as an important basis for the direction of any master data project. This project begins with an intensive analysis of the current situation, in which the cbs MDV tool can be used, for example, to determine the use and characteristics of master data across systems and to uncover potential for harmonisation. In addition to quantitative analyses, qualitative methods are also important, for example to identify current pain points and consolidate different perspectives on the topic.
Based on this, a master data strategy is developed using a fit-gap analysis, which provides a defined plan for how the company should ideally collect, manage, utilise and distribute certain master data in the system landscape in the future. cbs has developed its own process model based on so-called “building blocks”, which can make up a comprehensive master data strategy. In addition to technical aspects, governance and organisation are also taken into account. These building blocks are selected according to customer requirements and project-specific circumstances. In order to organise the project efficiently, it is also important to have a priority matrix that focuses on specific master data objects.
Keep data clean: maintaining a high level of master data quality
Every master data cleansing initiative should also consider the future perspective in order to ensure that high-quality master data continues to be maintained in accordance with the defined criteria. The target image is a “data-driven mindset” throughout the entire organisation, i.e. a shared awareness of the added value that data quality offers and that data governance is not a stumbling block, but rather enables secure and smooth processes in the first place.
Ideally, this can take place with the support of tools, as functionalities such as the integrated duplicate check, automatic derivation of field values or a validation check relieve the burden on users. The philosophy of master data governance is realised through the rule-based workflow, in which data is only activated after an appropriate check. The data maintenance process follows the “first time right” principle: the rules and checks make tedious reworking of master records obsolete and the data is immediately and correctly available.
The SAP MDG (Master Data Governance) tool, which we frequently implement in our projects, offers these and many other functionalities. The tool is not an end in itself; an important prerequisite is that there is a defined target image for both an organisational structure and a process organisation. Only if master data quality is seen as a continuous activity and an important contribution to value creation will the projects be successful in the long term.
Would you like to find out more about how you can establish effective and sustainable master data management in your company? We look forward to exchanging ideas with you!
Author
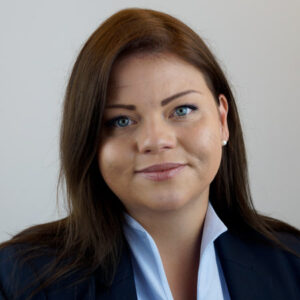